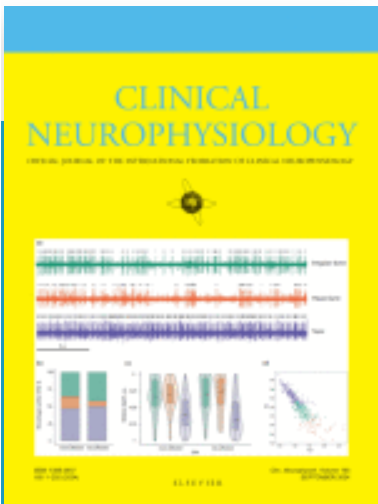
Clinical Neurophysiology : official journal of the International Federation of Clinical Neurophysiology
2024 Aug 5:166:152-165. doi: 10.1016/j.clinph.2024.07.018. Online ahead of print.
Abstract
Objective: To assess the value of combining brain and autonomic measures to discriminate the subjective perception of pain from other sensory-cognitive activations.
Methods: 20 healthy individuals received 2 types of tonic painful stimulation delivered to the hand: electrical stimuli and immersion in 10 Celsius degree (°C) water, which were contrasted with non-painful immersion in 15 °C water, and stressful cognitive testing. High-density electroencephalography (EEG) and autonomic measures (pupillary, electrodermal and cardiovascular) were continuously recorded, and the accuracy of pain detection based on combinations of electrophysiological features was assessed using machine learning procedures.
Results: Painful stimuli induced a significant decrease in contralateral EEG alpha power. Cardiac, electrodermal and pupillary reactivities occurred in both painful and stressful conditions. Classification models, trained on leave-one-out cross-validation folds, showed low accuracy (61-73%) of cortical and autonomic features taken independently, while their combination significantly improved accuracy to 93% in individual reports.
Conclusions: Changes in cortical oscillations reflecting somatosensory salience and autonomic changes reflecting arousal can be triggered by many activating signals other than pain; conversely, the simultaneous occurrence of somatosensory activation plus strong autonomic arousal has great probability of reflecting pain uniquely.
Significance: Combining changes in cortical and autonomic reactivities appears critical to derive accurate indexes of acute pain perception.
Keywords: Blood pressure; Classification models; Electroencephalography; Machine learning; Pain perception; Pupillary diameter; RR intervals; Skin conductance; Tonic pain.
Copyright © 2024 International Federation of Clinical Neurophysiology. Published by Elsevier B.V. All rights reserved.
- PMID: 39178550
- DOI: 10.1016/j.clinph.2024.07.018